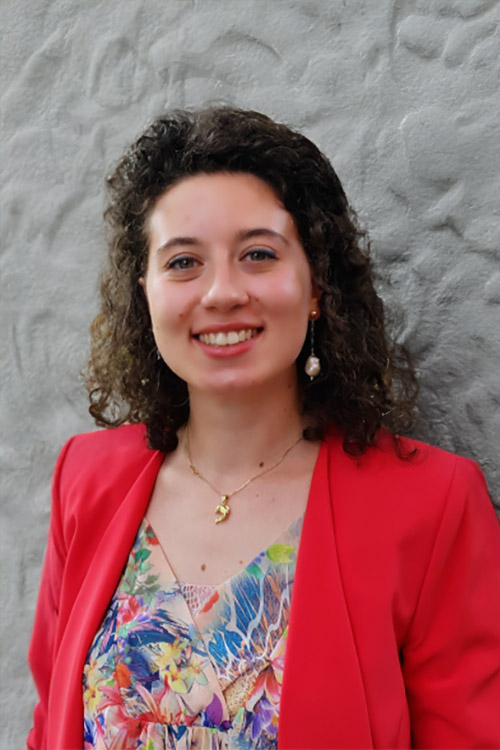
ORCID ID: 0000-0001-8269-2220
Curriculum: Experimental Neuroscience
Tutor: Professor Rosa Maria Moresco
Supervisors: Dr Elisabetta De Bernardi, Professor Gianpaolo Basso
Workplace: School of Medicine and Surgery – Monza U8 (Nanoscan7T MRI) and U28 (office) buildings; Neuroradiology Unit – Fondazione IRCCS San Gerardo dei Tintori Monza (Italy)
Abroad period: Mediso Medical Imaging Systems – Budapest, Hungary
I’m a Biomedical Engineer, graduated in July 2019 at Politecnico di Milano. I obtained my M.Sc. in the Technologies for Electronics field, with a thesis titled “A cooperative Serious Game supported by robotic therapy for stroke rehabilitation” (Final grade: 110/110 cum laude), under the supervision of Prof. Alessandra Pedrocchi, in collaboration with Villa Beretta – Presidio di Riabilitazione dell’Ospedale Valduce ~ Costa Masnaga (LC).
I’m currently enrolled in the PhD Program of Experimental Neuroscience at University of Milano-Bicocca, where in 2022 I held a position as Research Fellow. I focused on pre-processing, segmentation and analysis protocols for 18F-FDG PET imaging and 3T-MRI, considering patients with Alzheimer’s Disease and Mild Cognitive Impairment diagnosis, and patients surgically treated for brain tumor (Glioblastoma). With my current PhD project, I’m improving and adding further knowledge on fellowship topics.
From 2019 to 2022, I worked as Biomedical Research Engineer at Experimental Laboratory of Neuromotor Rehabilitation Researches, Istituto Auxologico Italiano (Milan, Italy) – Casa di Cura Capitanio. Under the direction of Prof. Luigi Tesio, I conducted translational research in gait and movement analysis, voluntary activation measurements and cervical proprioception analysis, focusing on neuromotor diseases, such as Multiple Sclerosis and Steinert Myotonic Dystrophy. Used instrumentation: Split-Belt Treadmill, Isokinetic Dynamometer, Optoelectronic System.
From 2020 to 2021, I attended the Master «RehabTech – Tecnologie per l’innovazione in medicina riabilitativa e per l’assistenza», at Politecnico di Milano (www.rehabtech.polimi.it), in collaboration with IRCCS Fondazione Don Carlo Gnocchi Onlus” ~ Milano. My final thesis focused on “Development of a Graphical User Interface for automatic analysis of MRI tractography applied to Disorder of Consciousness cases”.
In 2016, I obtained a Bachelor’s degree in Biomedical Engineering at Politecnico di Milano. My thesis, titled “Diagnosi precoce di danni neurologici tramite l’analisi quantitativa dei pattern di movimento degli arti nei neonati” (i.e. “Early diagnosis of neurological damage by quantitative analysis of limb movement patterns in infants”), was conducted under the supervision of Prof. Raffaele Dellacà, in collaboration with Fondazione IRCCS Cà Granda Ospedale Maggiore Policlinico di Milano.
Professional skills:
- Programming languages: Matlab | Basic Python | C | C++ | C# | LAteX
- Nanoscan7T preclinical MRI: MRI Acquisition through NuclineTM software, Processing through InterViewTM FUSION software
- Software and Tools: Statistical Parametric Mapping (SPM12) | TrackVis | Diffusion Toolkit | Slicer3D | ITK-SNAP | FreeSurfer | FSL | DeepBraTumIA | Unity 3D | Visual Studio | BTS Bioengineering Smart Suite (Capture, Tracker, Analyzer) | SPSS software | STATA | Basic knowledge in EEGLab | OpenSim | SigmaPlot | Basic knowledge of Solidworks
- Languages: Level B2 in English (TOEIC exam) and German (Goethe-Institut Zertifikat)
Besides scientific publications, I submitted abstracts for posters and oral presentations at 31st AINR congress (2022), VI congresso AINR funzionale (2022), 36th and 35th EANM congresses (2022-2023), 19th EMIM congress (2024) and NeuroMI congresses (2022-2023). I attended the “MRI Pulse Sequences – Principles, Misconceptions & Applications” workshop at EMIM (2024) and the “RADIOMICS TOOLBOX WORKFLOW AND QUALITY MANAGEMENT” workshop at Fondazione IRCCS Policlinico San Matteo, at Mondino Foundation – Pavia (2022).
PhD research project
Development of pathology-specific tools for multimodal neuroimaging quantitative analysis and modelling
Background: The integrated quantitative analysis and modeling of structural and functional information provided by multimodal neuroimaging techniques (i.e., PET, MRI) may help addressing open clinical/research questions and understanding human brain functional organization thus impacting the way medicine is performed. Multimodal neuroimaging requires the development of application-specific tools and models to face spatial registration, segmentation and quantification issues. Depending on image kind and characteristics and on the clinical/research question, customization of both image preprocessing and quantification/modeling approaches has to be addressed.
Aim: The aim of the project is to develop quantitative tools for neuroimaging analysis and modeling, to support the clinical practice in patient classification, diagnosis, and prognosis understanding. Processing/analysis pipelines and models will be declined and optimized depending on pathology under investigation, image type (PET or MRI), image characteristics (contrast, spatial resolution and noise), and analyzed outcome. Developed tools and models will be furthermore linked with preclinical models, with the aim to transpose tools and knowledge as much as possible from one field to the other.
Materials and Methods: Neuroimaging processing and analysis will be structured according to the following general steps. 1. Acquisition step: Focalization on images acquisition, parameters tuning, motion and artifacts correction methods. Selection of relevant available acquired modalities. 2. Preprocessing step. Spatial co-registration within and inter modalities, to allow the precise localization of corresponding areas among imaging techniques or multiple scans (e.g. or when dealing with pre-post-follow up acquisitions). Spatial normalization in a common reference space. Image quality control to improve signal by means of different correction methods. 3. Region of interest (ROIs) and Volumes of interest (VOIs). Image segmentation through already developed tools or through customized scripts. 4. Analysis and model definition. Quantitative statistics analysis by single-subject, by group or single subject vs group analysis. Correlation studies between modalities. Radiomic analysis, and consequent definition of predictive and classification machine learning models. Customized analysis routines. These four general steps will be developed and adapted, according to the specific application field.
First results and discussion: First application regards glioblastomas field. A database of surgically treated patients have been considered. Multiple weight preoperative MR sequences (T1, T2, FLAIR and contrast-enhanced T1) acquired with heterogeneous protocols have been used to segment tumor core and T2-FLAIR hyper intensity through a deep learning tool. A specific operative structure for the two ROIs evaluation has been set, relying on a senior neuroradiologist experience, accounting for images characteristics and considered tumor typology. Automatic segmentation performances are assessed as a support for neurosurgeons, discerning its advantages and limitations in a surgical planning. Second application regards the cognitive neuroscience field, in particular the study of the Mild Cognitive Impairment status. A first specific purpose was to define an optimized protocol of preprocessing and combined multi-techniques analysis to investigate correlation between metabolism (18F-FDG PET) and perfusion (pCASL), to build models to understand neurodegenerative pattern, exploiting both metabolic and perfusion information. The definition of pattern that can characterize MCI patients can be modeled and correlate with clinical outcomes and in a prospective way will be used to predict potential progression to neurodegenerative disease already at the MCI stage. Moreover, metabolism analysis could become a driver to interpret perfusion imaging results and perfusion data could be added to clinical biomarkers as useful indices for diagnosis and prediction. A ROI-based quantitative pipeline for joint analysis has been developed and already validated on its PET line, relying on available PET reference data. Further correlation and cluster analysis are ongoing to investigated relation of metabolic and perfusion information.A third project’s application regards the preclinical MRI sequence optimization. In particular, phantom acquisitions performed on a Mediso nanoScan7T MRI have led to preliminary results based on a Signal to Noise Ratio (SNR) improvement. The focus was on parameters optimization of advanced diffusion MRI sequence (i.e., Diffusion Weighted Imaging and Diffusion Tensor Imaging – DWI/DTI).
Additional details
To get further details please visit:
-
Scopus Author ID: 57223406891
-
Research Gate profile:
-
Web of Science ResearcherID: AAX-5400-2021